What does it take to make AI truly multilingual? In this interview, we speak with Romina Oji, a PhD student at Linköping University, who is helping reshape how language models handle underrepresented languages. From adapting smaller models to sound more natural in low-resource settings, to advancing fairness and inclusivity in AI, her work highlights how smarter techniques can lead to more equitable language technology.
What are you currently working on in the TrustLLM project?
I’m currently contributing to Work Package 4 (WP4) of the TrustLLM project, where I focus on building and evaluating language-specific adapters for multilingual large language models. The core of my work involves adapting smaller, English-centric models to better understand and generate text in under-resourced languages, specifically, the Germanic languages that TrustLLM is centered around: Icelandic, Faroese, Danish, Swedish, and Norwegian.
Why does this matter? Most language models today are heavily skewed toward English, and smaller models in particular tend to struggle when it comes to handling languages that weren’t well represented during training. That’s where we come in. Our goal is to make these smaller models more linguistically inclusive, without requiring massive amounts of new data or computing resources.
Which challenges do you find most interesting to solve through the project?
One of the most interesting challenges for me is figuring out how to make small language models work well in languages that have limited training data available. It’s easy to get good results in English or other high-resource languages, but things get much trickier when you’re dealing with very low-resource languages like Icelandic or Faroese, where there just isn’t as much data to work with. I find it fascinating to explore how far we can push these models using adaptation techniques, rather than relying on massive compute or retraining everything from scratch.
Another challenge I really enjoy is making sure the models don’t just function in a language, they actually sound like they belong in it. It’s not just about getting the meaning right or following grammar rules. It’s about whether the phrasing feels natural, whether it flows the way a native speaker would write or talk. Each language has its own personality, and helping the models pick up on that is a really fun and satisfying challenge. When I see one of our models generate something that just feels right in a language like Icelandic or Faroese, it’s such a rewarding moment. It means we’re not just building smarter models, we’re making them more respectful of the languages they’re learning.
And of course, there’s the broader challenge of inclusivity. It’s motivating to work on a project where the goal isn’t just performance, but fairness and representation, bringing better language support to communities that haven’t had much voice in the AI space so far. That’s a challenge worth solving.
What has been the most inspiring experience during your work on the project so far?
Beyond the technical milestones, what’s been most inspiring is being part of a project that brings together such a wide range of people, all with different expertise and a shared purpose. TrustLLM isn’t just about training better models; it’s about rethinking how we approach language technology as a whole. There are researchers focused on core model architecture, others working on high-quality data collection, experts in evaluation and alignment, and people thinking deeply about fairness, transparency, and ethical AI. Everyone contributes a unique piece of the puzzle, and it’s this kind of interdisciplinary teamwork that really drives the project forward.
At the same time, what makes the work so personally meaningful is that the project genuinely prioritizes linguistic diversity and inclusion. It’s not just about building smarter models—it’s about making sure people who speak these languages are part of the AI future. That bigger purpose adds a level of depth to the work that goes far beyond the code or the experiments. It’s about creating technology that reflects and respects the wide range of human language.
What are you most looking forward to achieving in the coming months?
One thing I’m really looking forward to is the Second Annual Consortium Meeting of TrustLLM in Iceland in June. Even though we have regular weekly and monthly meetings, it can still be hard to get a full picture of what’s happening across the different work packages. A lot of the time, we’re all deep in our own tasks, and we only get glimpses of the bigger picture. The upcoming meeting will be a great opportunity to connect with other teams in person and really understand how all the pieces of the project fit together. I’m especially excited to learn more about the parts of the project I haven’t been as directly involved in, like alignment techniques and evaluation. Having those in-depth conversations face-to-face makes it easier to share insights, ask the right questions, and spot connections between our workstreams that aren’t always obvious in virtual meetings.
How do you think the TrustLLM project could impact the AI field?
One of the things that makes this project really special is its commitment to giving underrepresented languages the attention they deserve. We’re not just fine-tuning models for the sake of benchmarking; we’re trying to build tools that people can actually use, in the languages they speak every day. That kind of focus on real-world use makes a huge difference. It’s about making sure language technology doesn’t just work for the few, but for everyone. With thoughtful methods and a focus on real-world impact, we can build language models that are not just powerful, but truly respectful of the wide range of human language.
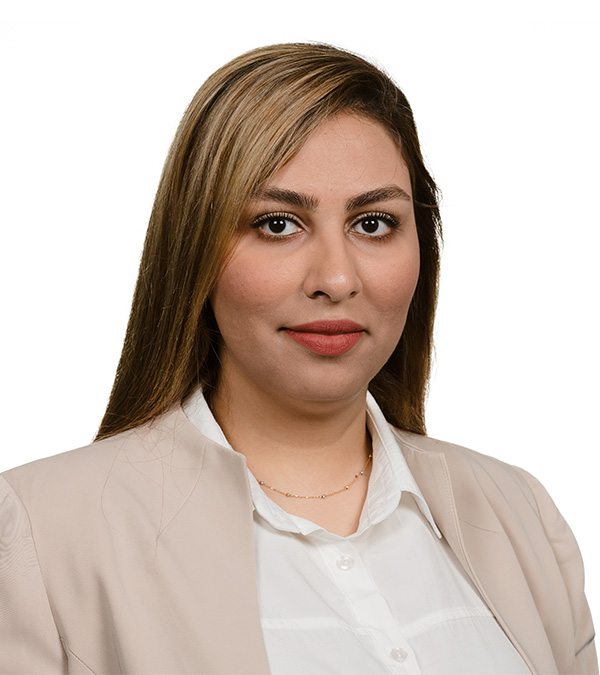
Romina Oji, PhD student, Linköping University